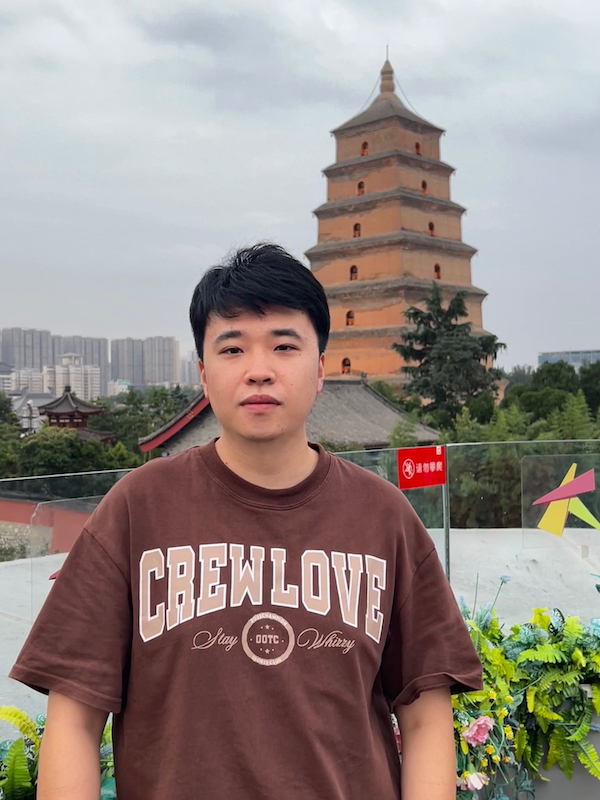
Xinyang Liu (刘昕洋)
Master student
School of Electronic Engineering
Xidian University
Email: xinyangATK [AT] gmail [dot] com
Google scholar | Github | Twitter
Bio
Howdy! I am a research intern at Purdue University, advised by Prof. Ruqi Zhang. I’m also work closely with Prof. Mingyuan Zhou, an Associate Professor at the University of Texas at Austin. I received my M.S degree from Xidian University in 2024, advised by Prof. Bo Chen. Previously, I obtained my B.S degree from Xidian University in 2021.
My research interests lie in the general area of machine learning, particularly in solving real-world problems through advanced Generative AI systems. My recent research focuses on Generative Modeling, including its theoretical exploration and various applications in data generation and multimodal learning.
Now I’m looking for PhD 25 Fall and here is my CV !
In addition, I am also highly interested in 2D & 3D generation, robot learning, planning, and agent learning upon Generative AI.
If you share the same research interests with me, feel free to reach out or add my WeChat.
news
Jan, 2025 | Happy Birthday🍰🕯️👑 The best gifts come from two accepted papers! Much appreciation to all of my collaborators and advisors! In “Optimal Stochastic Trace Estimation in Generative Modeling” (AISTATS 2025), we leverage the Hutch++ estimator in generative modeling and propose a practical algorithm that amortizes decompositions to reduce costs, while also providing theoretical guarantees specifically in generative modeling context. In “Advancing Graph Generation through Beta Diffusion” (ICLR 2025), we futher explore the potential of Beta Diffusion in graph modeling and propose a novel graph-driven generative process with concentration modulation technique, which makes Beta Diffusion unique again! |
---|---|
Jun, 2024 | I graduated with a master’s degree in Xidian University! |
Apr, 2024 | Our paper “Patch-Prompt Aligned Bayesian Prompt Tuning for Vision-Language Models” is accepted by UAI 2024. |
Sep, 2023 | Two papers are accepted by NeurIPS 2023! |
Apr, 2023 | Our paper “Bayesian Progressive Deep Topic Model with Knowledge Informed Textual Data Coarsening Process” is accepted by ICML 2023. |
Feb, 2023 | ![]() ![]() ![]() PyDPM is a python library focuses on constructing Deep Probabilistic Models (DPMs). Our developed Pydpm not only provides efficient distribution sampling functions on GPU, but also has included the implementations of existing popular DPMs. |
selected publications
(*) denotes equal contribution